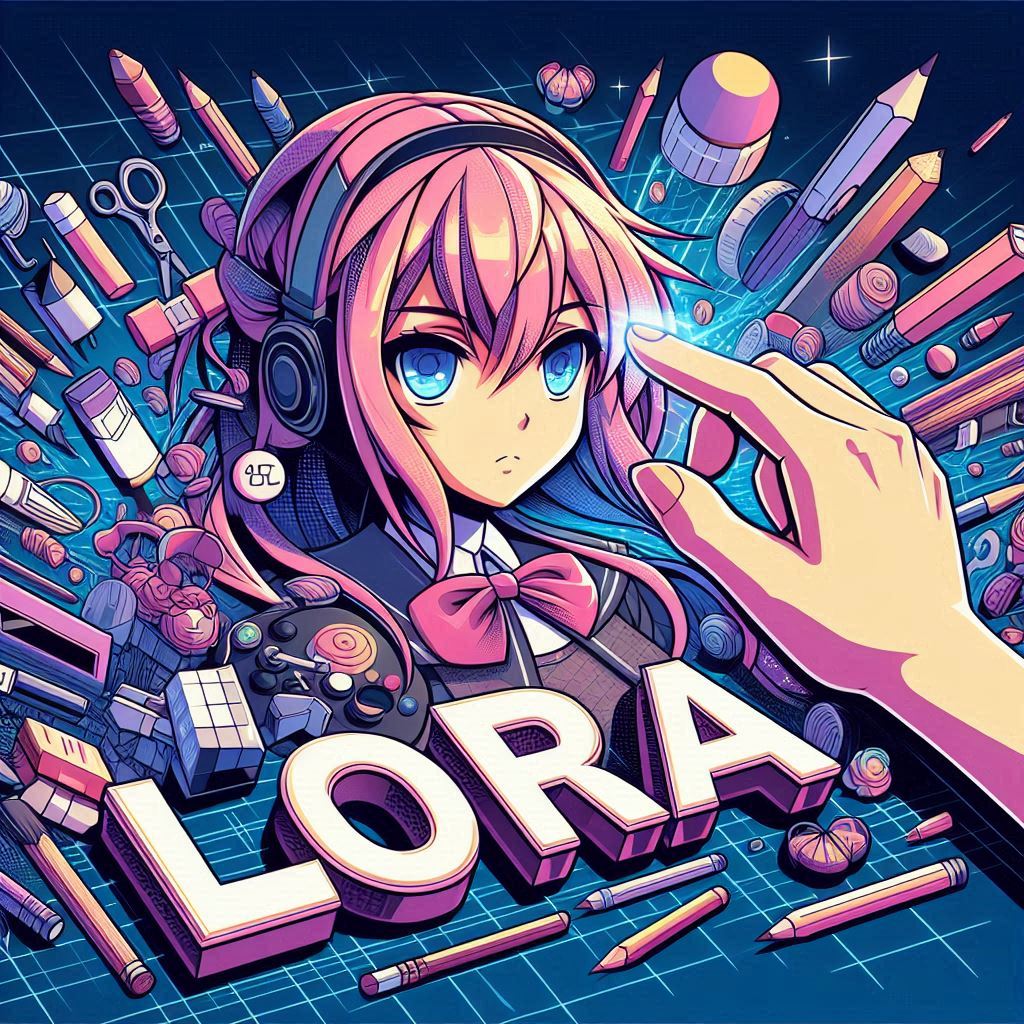
Among the myriad of techniques that drive AI image-generating platforms, Low-Rank Adaptation (LoRA) stands out as a particularly crucial and effective method.
Understanding LoRA: The Fundamentals
Low-Rank Adaptation is a technique that aims to reduce large-scale models' complexity. This decomposition allows for efficient storage and computation, which is particularly valuable in the AI image generation context, where models often require substantial computational resources.
Improved Model Adaptability
One of the challenges in AI image generation is the need to adapt models to different styles, themes, and user preferences. LoRA facilitates this adaptability through efficient fine-tuning and transfer learning.
Style Transfer: LoRA can fine-tune pre-trained models to generate images in specific artistic styles or adapt to the visual themes needed. This is achieved without extensive retraining, thanks to the reduced parameter space.
Note: The all of the image below has the same prompts and the parameters except the style-related LoRA
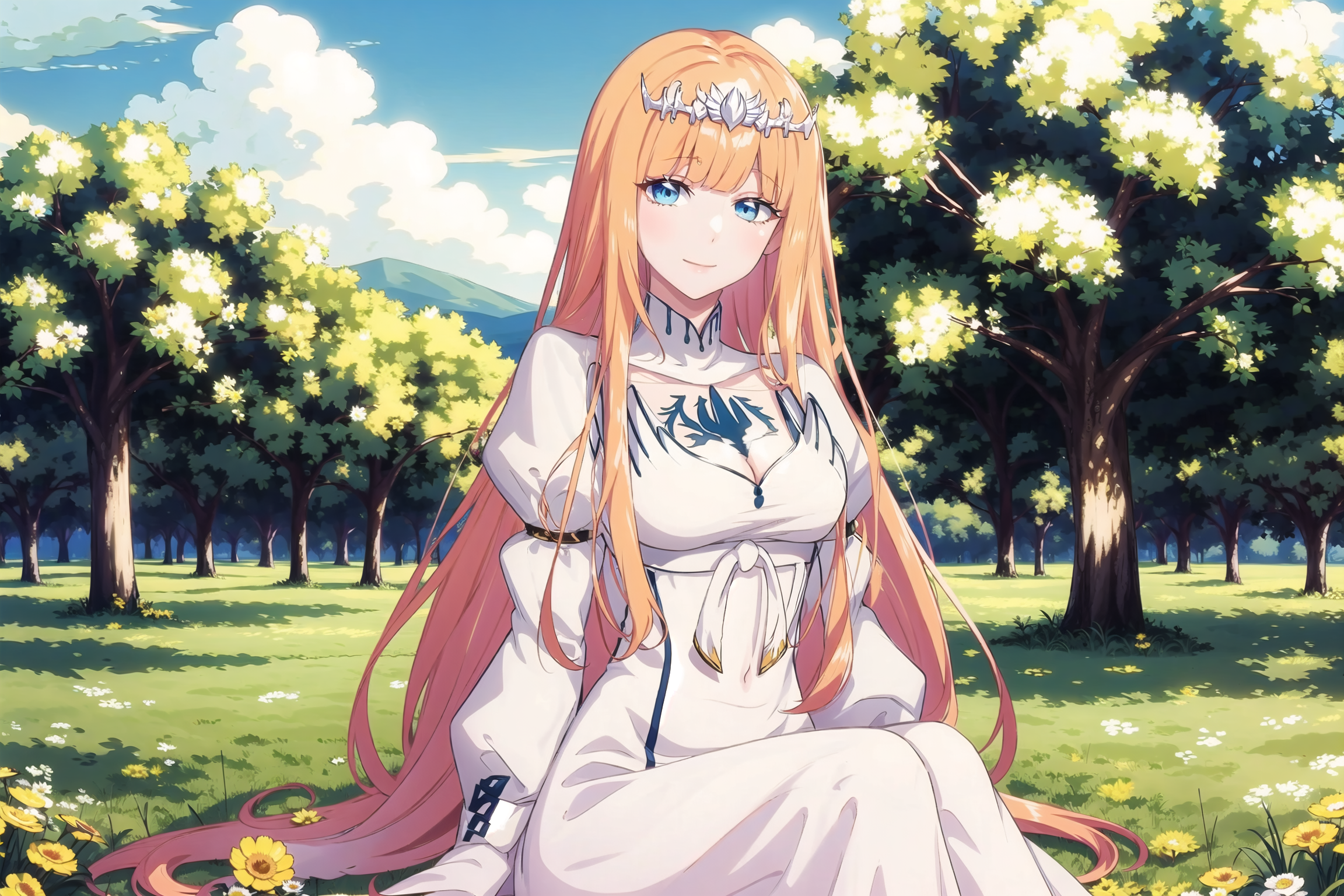
.......CalicoMix FlatAni - v1.0 without any other LoRAs apart from the one for my waifu (Calca Bessarez)...........
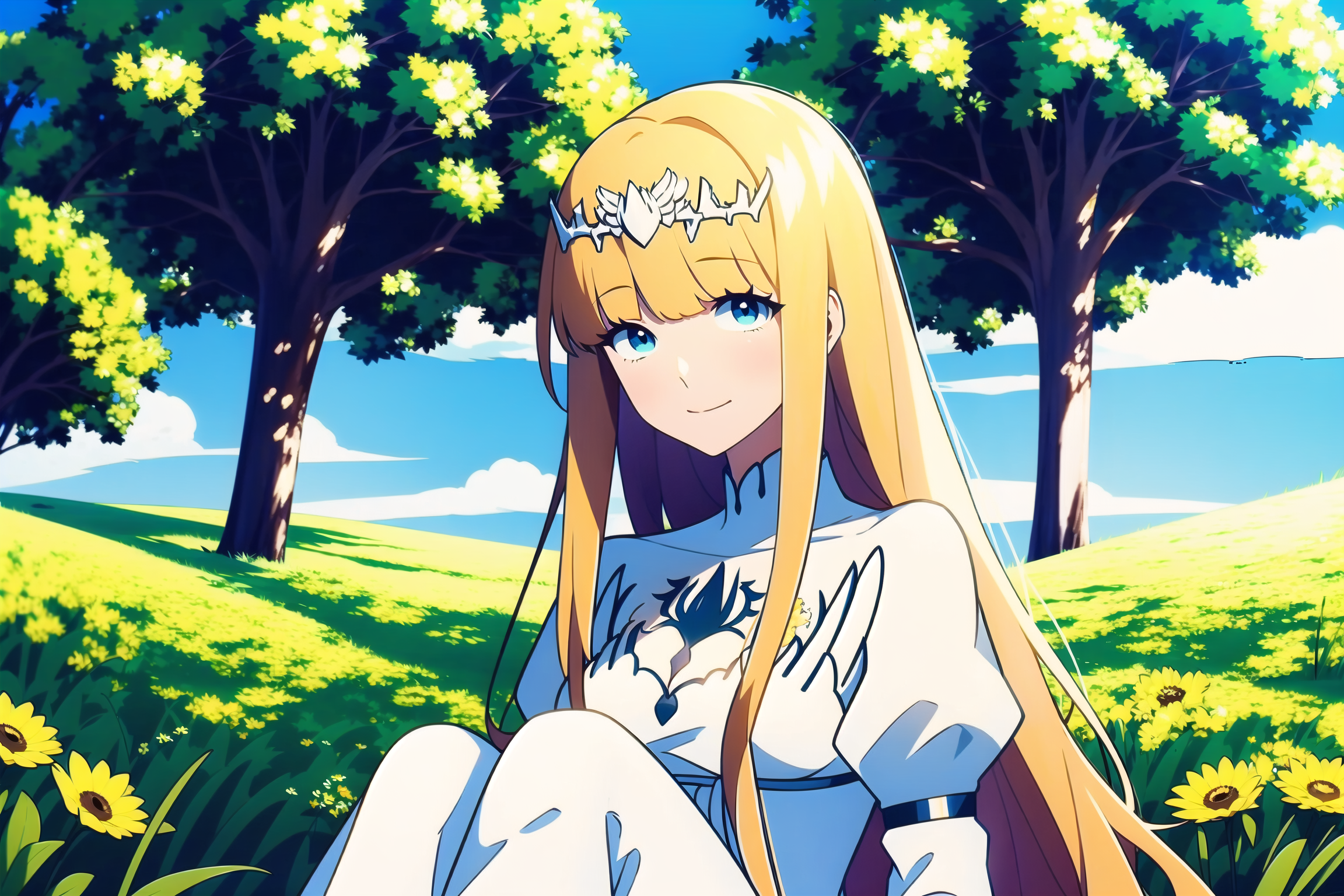
....................................................CalicoMix FlatAni - v1.0 with DesillusionRGB as an extra LoRA....................................................
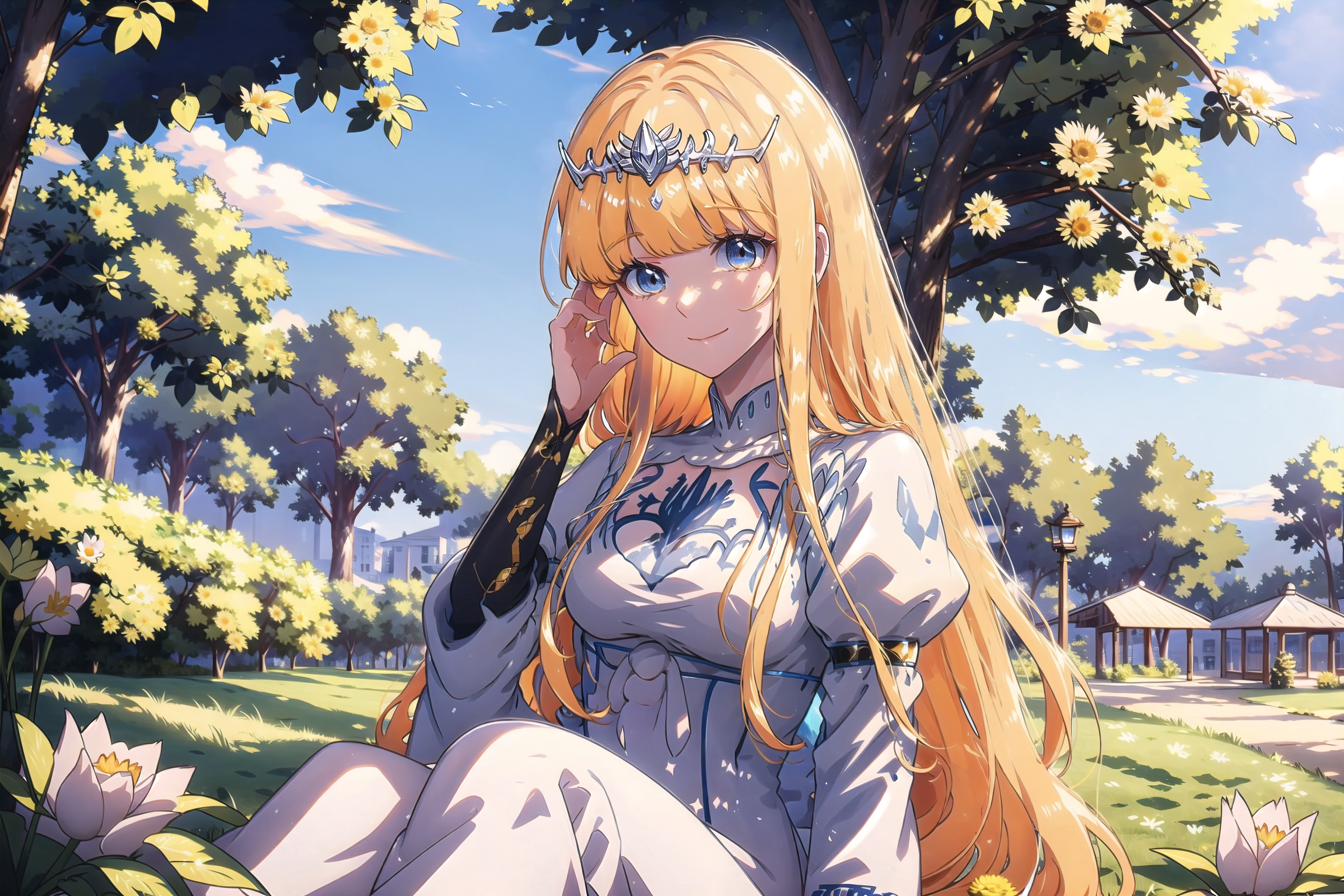
......................................CalicoMix FlatAni - v1.0 with Glitter and Shiny details as an extra LoRA.......................................
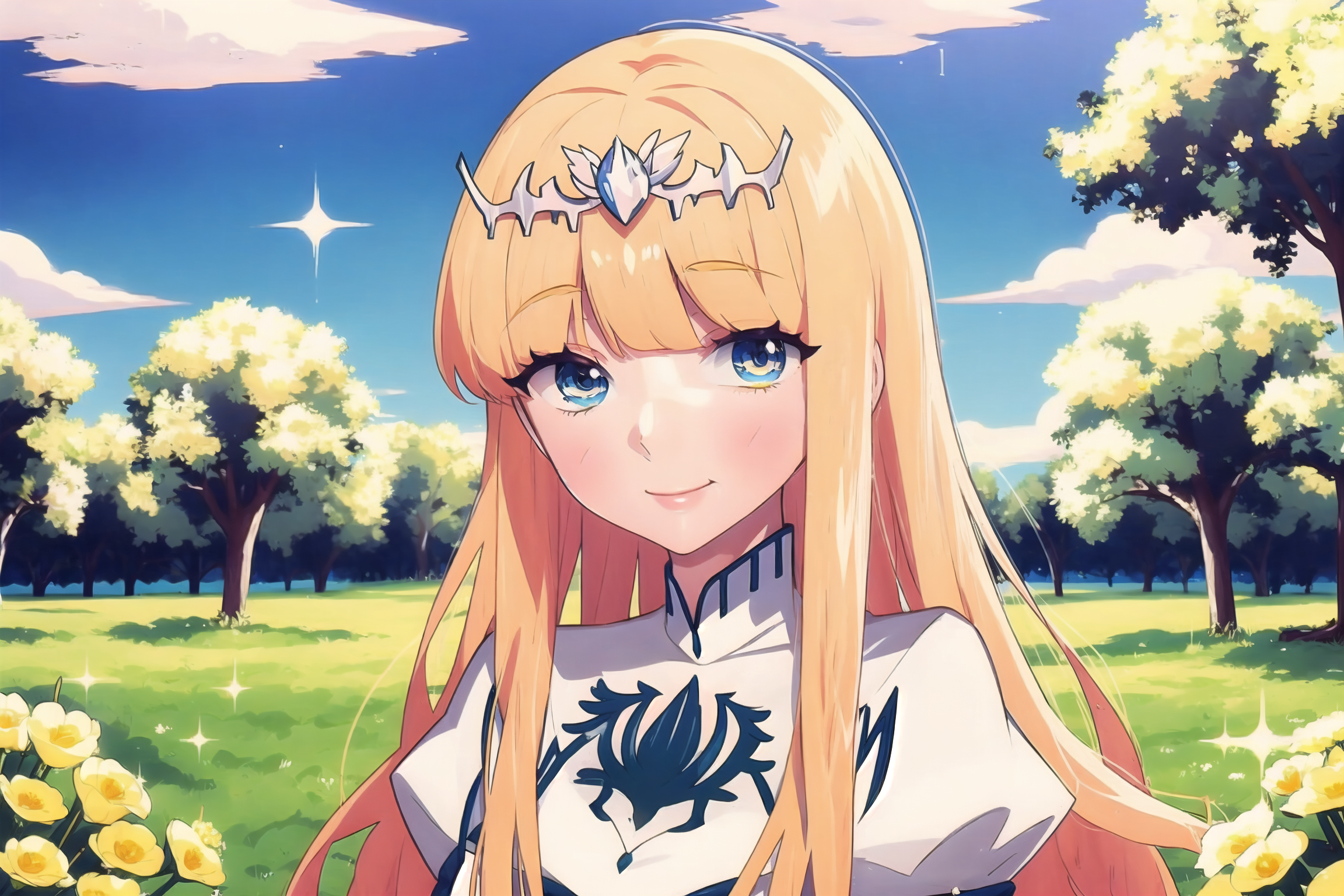
..........................................CalicoMix FlatAni - v1.0 with Retro Lofi - Pop Art (Style) - v2.0 as an extra LoRA.....................................
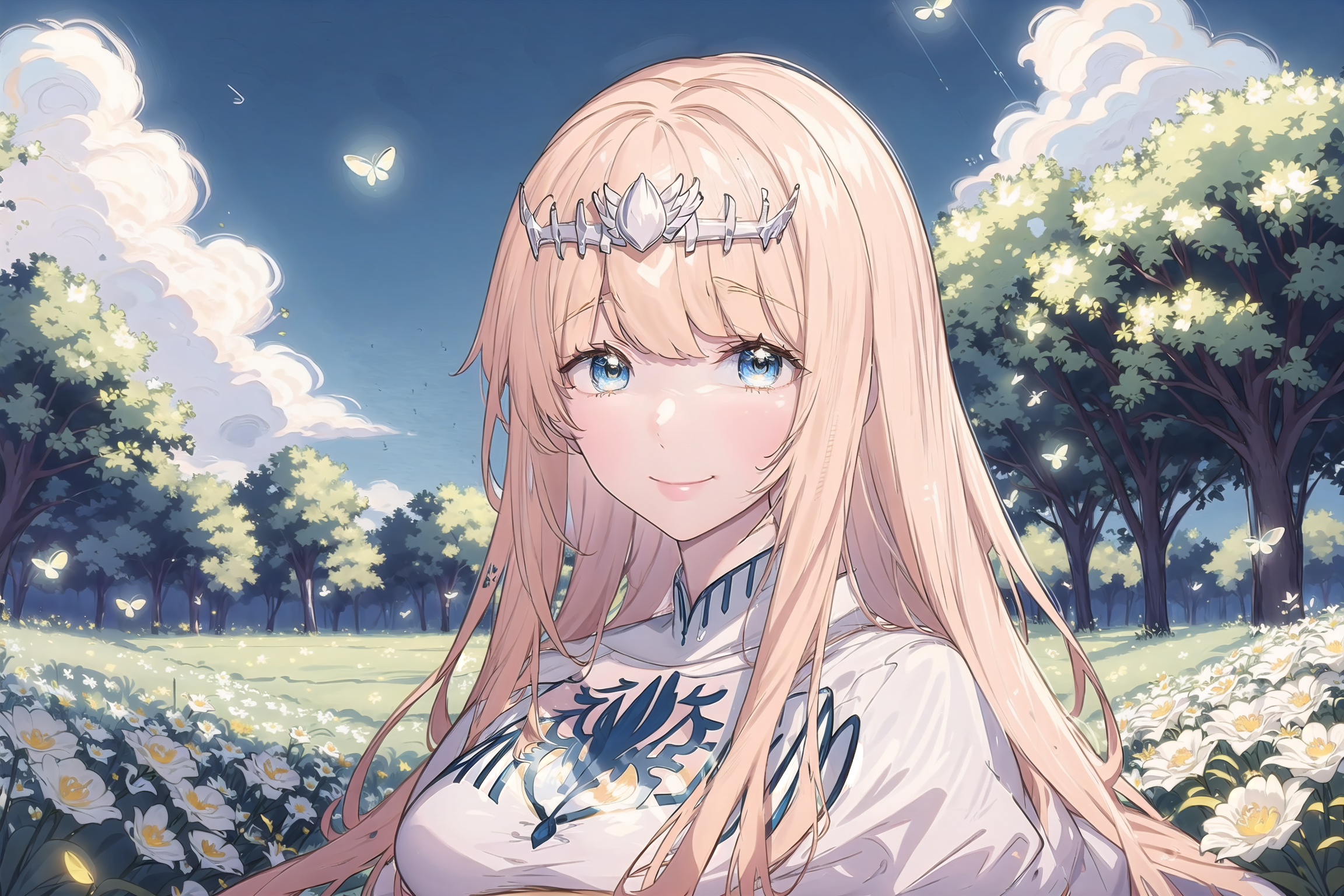
..................................................CalicoMix FlatAni - v1.0 with Fireflies ホタル as an extra LoRA.............................................
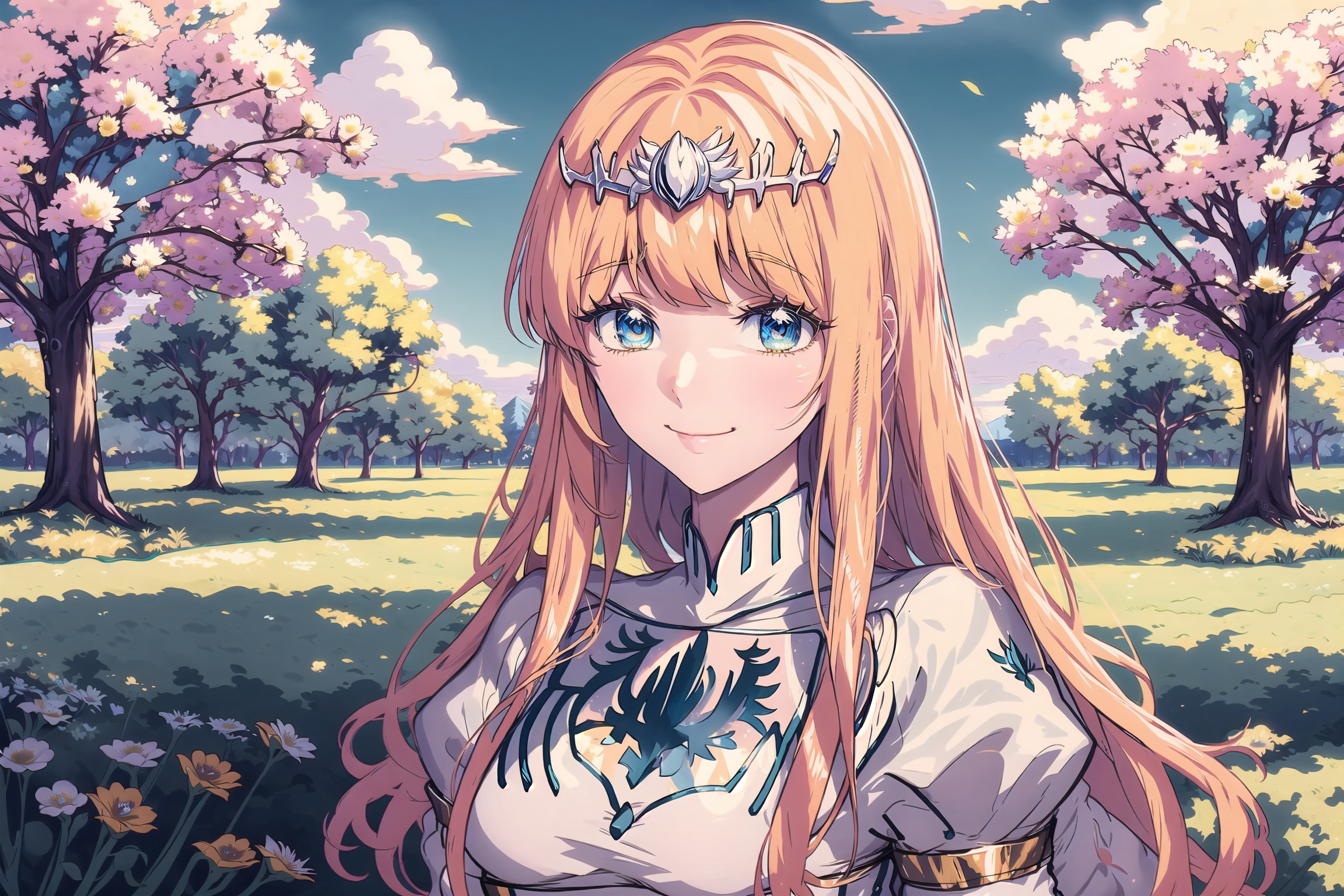
..............................................CalicoMix FlatAni - v1.0 with tshee - vector style art - v1.0 as an extra LoRA.....................................
Personalization: Users can personalize image generation by training models on their datasets or preferences, especially for specific art styles, sceneries, or characters. LoRA enables these customizations to be performed quickly and efficiently, enhancing user satisfaction and engagement like Calca Bessarez in the example above.
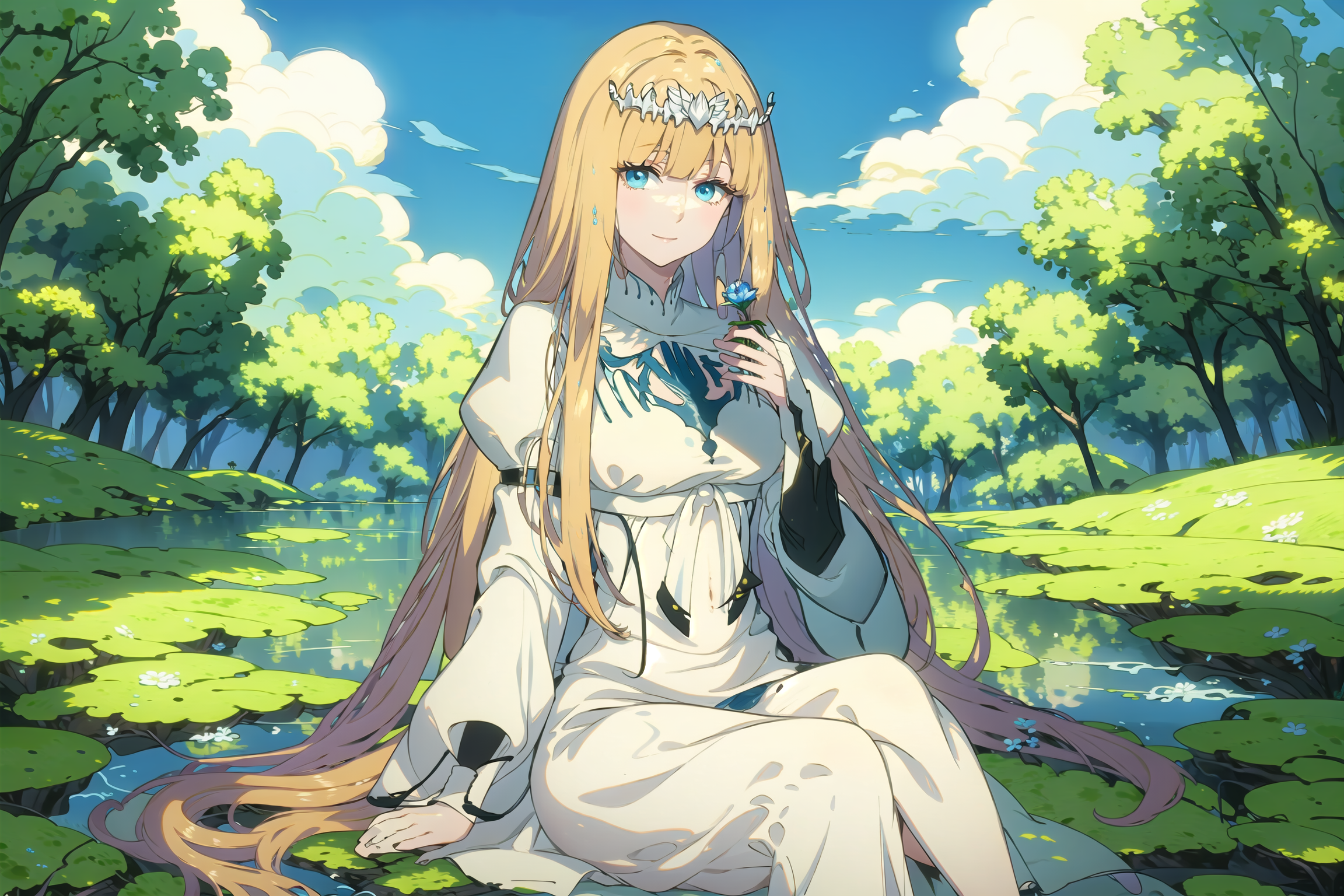
.....................................CalicoMix FlatAni - v1.0 with Swamp / Giant Tree Forest 绪儿-巨树森林背景 - XRYCJ as an extra LoRA.....................................
However, in the case of eliminating variation, customization of the outfit, or changing the character's physical appearance, the method of weighting parameters needs to be considered, from the default value of 0.8 to 1.0 (similar to the original design), above 1.0+++ (look very the same to the original design), lower than 0.5 (not pay attention to the original design)
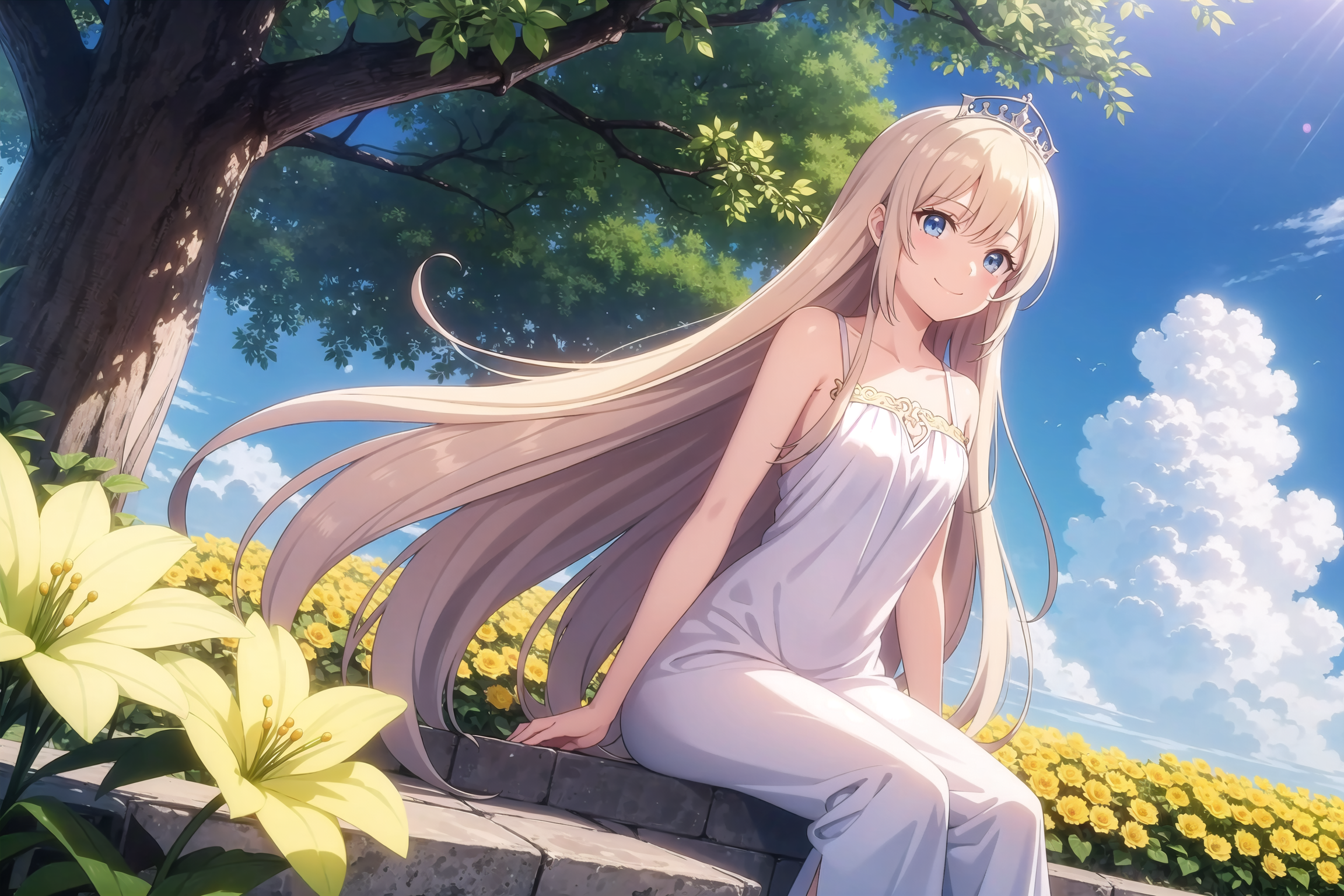
........CalicoMix FlatAni - v1.0 without any LoRA even the one for the character design
So the result for the same prompt is just an ordinary girl.......
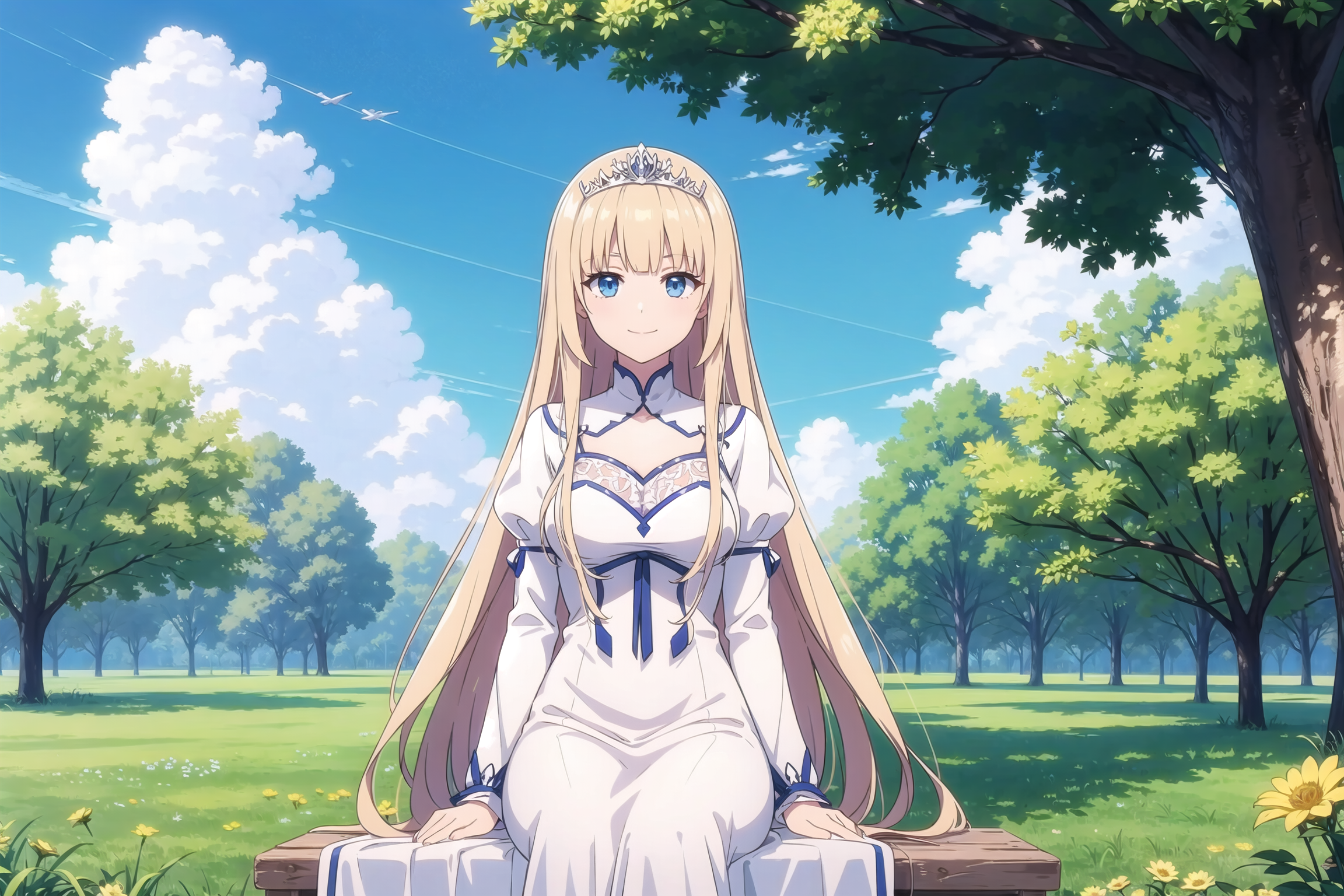
.................................................CalicoMix FlatAni - v1.0 with Calca's LoRA but weighting with 0.3.....................................
.............................................(There is no kingdom symbol on her chest and the tiara's shape is not the original one).....................................
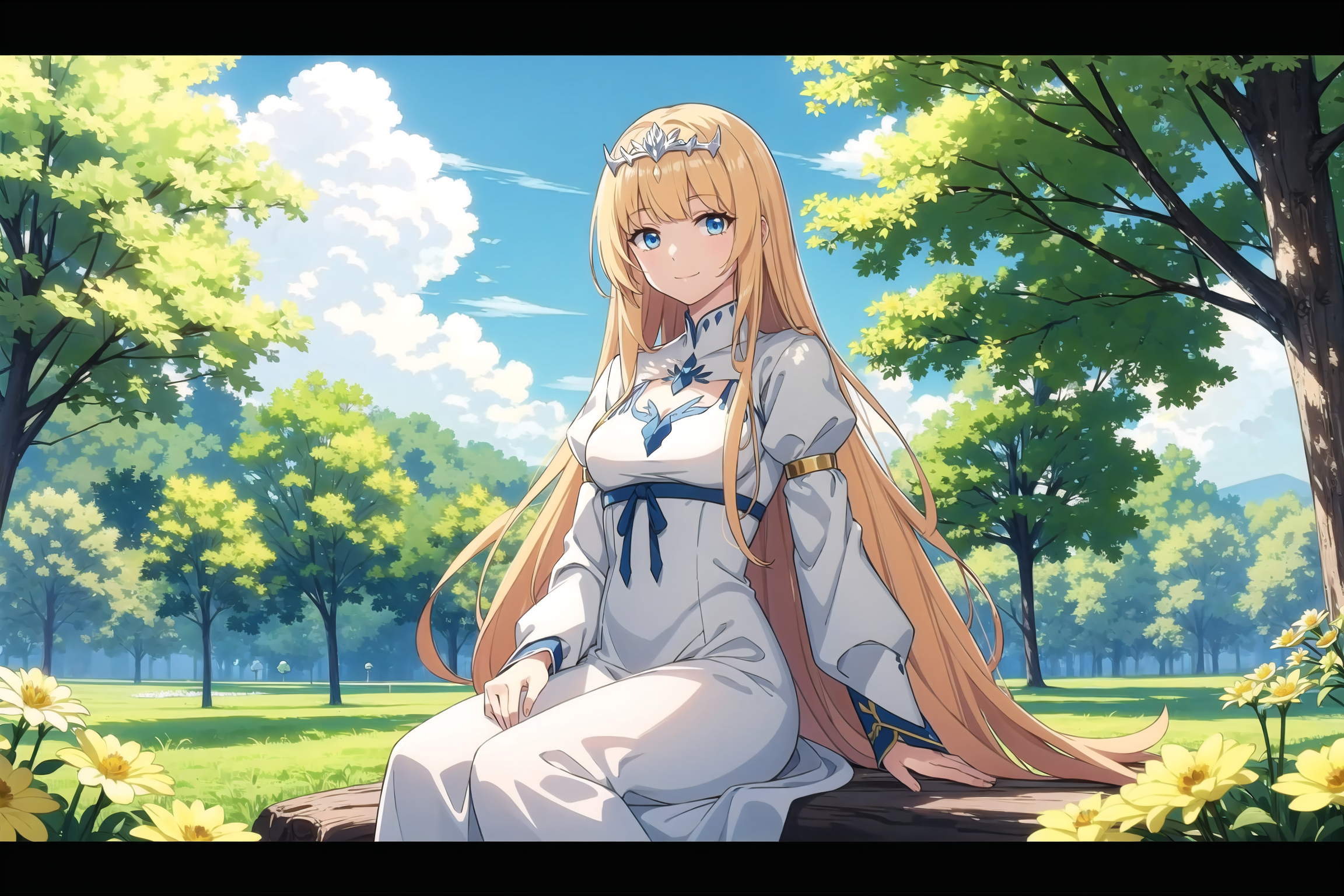
..................................................CalicoMix FlatAni - v1.0 with Calca's LoRA but weighting with 0.5.....................................
.....................................(Her dress and tiara are very close to the original, but there is no kingdom symbol on the chest)...........................................
A key limitation
Although Low-Rank Adaptation (LoRA) offers significant benefits in AI image-generating platforms, including reduced computational complexity and memory usage, it also comes with limitations that can impact its effectiveness and applicability. Here are some key limitations of LoRA in the context of AI image generation:
1) Approximation Errors
LoRA involves approximating a high-dimensional matrix with two lower-dimensional matrices. This approximation can introduce errors that affect the performance and quality of the model. Specifically: Loss of Detail and Bias in Representation
2) Model Compatibility and Integration
While LoRA is effective in many scenarios, integrating it into existing AI frameworks and models can present a challenge like Compatibility Issues as not all models are equally suited for low-rank approximations.
3) Scalability Limitations
Although LoRA helps reduce the computational load, there are still scenarios where scalability remains an issue:
Extremely Large Models: even though the reduced matrices can be substantial, the extremely large model still requires considerable computational resources and memory.
Real-Time Constraints: In applications demanding ultra-low latency, such as real-time image processing, the approximation process might still introduce unacceptable delays.
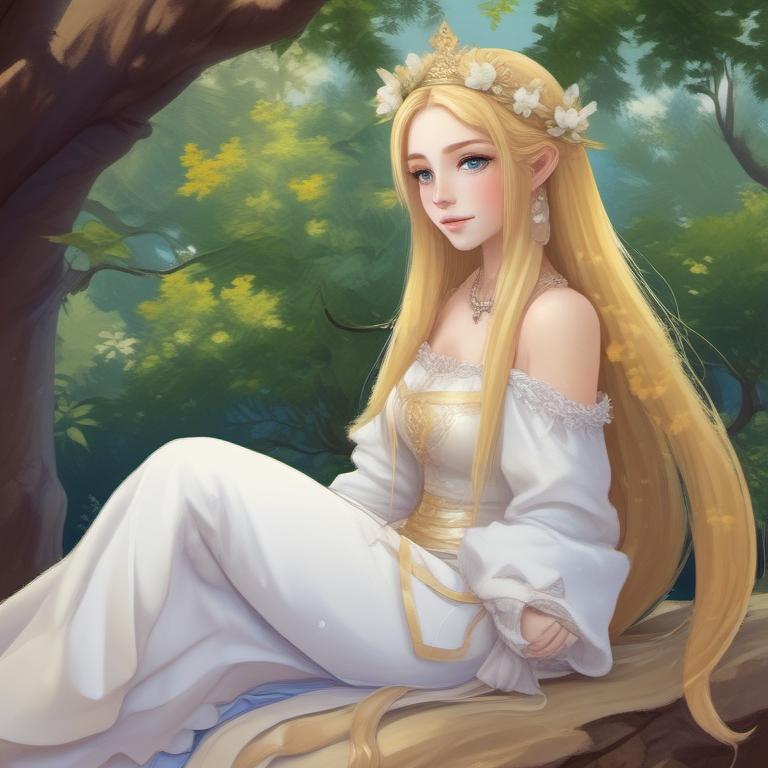
.......................................................Result of real-time generation from the same prompt I used in the previous examples...........................................
............................................In this case, even the main model, CalicoMix FlatAni - v1.0, is not available.....................................
4) Complexity and Availability of the platform
LoRA models, despite being optimized for efficiency, still consume computational resources such as memory and processing power. allowing an unlimited number of LoRA could:
Overwhelm System Resources: The computational demands of managing multiple LoRA models simultaneously could exceed the available system resources, leading to slower performance or crashes.
Increase Latency: Each additional LoRA model increases the complexity of the image generation process, potentially leading to higher latency and slower response times for users.
This might be a reason why many AI image-generating platforms limit the number of LoRA for one batch of images (3 for free users and 6 for pro users in the case of Tensor.Art)
.....................................Yeah, I'm not here to sell the subscription, but if you guys have no financial constraints, it would be very helpful to support our dedicated developers here in the Tensor.Art like myself. :P To enjoy the benefit of using 6 maximum LoRAs in total......................................