📢I am participating in the Project Odyssey - Season 2 AI Video Competition. If you are interested in my work, please vote for me. Your support would mean the world to my creations. Thank you so much! 🤝
🎧Music Video:https://civitai.com/images/51310889 👈👍❤️🫶
Introduction
In the realm of AI image generation, particularly with models like Pony Diffusion, achieving high-quality outputs consistently is a significant challenge. A crucial innovation to address this challenge involves the use of aesthetic ranking tags such as score_9, score_8_up, score_7_up, score_6_up, score_5_up, and score_4_up. These tags play a vital role in guiding the model to produce better images by leveraging human-like aesthetic judgments. This article delves into what these tags are, their purpose, and how they are utilized to enhance the quality of AI-generated images.
What Are Score Tags?
Score tags are annotations added to image captions during the training phase of AI models. These annotations indicate the aesthetic quality of the images, based on a scale derived from human ratings. Here is a breakdown of the specific tags:
1. Score_9: Represents the highest quality images, typically in the top 10% of all images.
2. Score_8_Up: Includes images that are in the top 20%, from 80% to 90% in quality.
3. Score_7_Up: Covers images in the top 30%, from 70% to 80% in quality.
4. Score_6_Up: Encompasses images in the top 40%, from 60% to 70% in quality.
5. Score_5_Up: Represents images in the top 50%, from 50% to 60% in quality.
6. Score_4_Up: Includes images in the top 60%, from 40% to 50% in quality.
These tags are used during the training of AI models to help the model distinguish between different levels of image quality, thereby enabling it to generate better images during the inference phase.
Purpose of Score Tags
Enhancing Model Training
The primary purpose of score tags is to improve the training process by providing the model with a clear understanding of what constitutes a good image. By repeatedly exposing the model to images annotated with these tags, it learns to recognize the characteristics that make an image aesthetically pleasing.
Providing Fine-Grained Control
Score tags offer fine-grained control over the quality of the generated images. Users can specify the desired quality level in their prompts, ensuring that the output meets their expectations. For example, using the score_9 tag in a prompt indicates that the user expects the highest quality images.
Overcoming Data Quality Challenges
In large datasets, not all images are of high quality. Score tags help in filtering out lower-quality images during the training phase, ensuring that the model is trained on the best possible data. This selective training helps in achieving better overall performance and higher quality outputs.
How Score Tags Are Used
Training Phase
During the training phase, images in the dataset are manually or semi-automatically annotated with score tags based on their aesthetic quality. This process involves:
1. Data Collection: Gathering a diverse set of images from various sources.
2. Manual Ranking: Expert reviewers rank the images on a scale, typically from 1 to 5, based on aesthetic criteria.
3. Tag Assignment: Images are tagged with the corresponding score tags (e.g., score_9 for top-tier images).
The model is then trained on this annotated dataset, learning to associate the score tags with the quality levels of the images.
Inference Phase
During the inference phase, users can include score tags in their prompts to influence the quality of the generated images. For example:
•A prompt with the tag score_9 will generate images that the model has learned to associate with the highest quality.
•A prompt with the tag score_6_up will generate images that meet the quality standards from 60% to 100%.
This tagging system provides users with the flexibility to request images of varying quality levels, depending on their specific needs.
Practical Application
In practice, the use of score tags can vary depending on the tools and interfaces available. Some tools, like the PSAI Discord bot, automatically add these tags to prompts, simplifying the process for users. In other interfaces, such as Auto1111, users may need to manually add these tags to their prompts. This can be done by saving the tags as a style or copying and pasting them into the beginning of the prompts.
Limitations and Future Improvements
While score tags significantly enhance the quality of AI-generated images, there are some limitations:
1. Bias in Tags: The tags can introduce biases, especially when using style or artist-specific LoRAs. This may affect the diversity and creativity of the outputs.
2. Negative Tags: Negative tags (e.g., score_4) are less effective because the training data does not include extremely low-quality images. Therefore, their impact on steering the model away from bad images is limited.
Future improvements for Pony Diffusion V7 aim to refine the tagging system and enhance the model’s ability to understand and utilize these tags effectively. Simplifying the tags and ensuring a more diverse training dataset are key areas of focus.
Conclusion
Score tags like score_9, score_8_up, score_7_up, score_6_up, score_5_up, and score_4_up play a crucial role in enhancing the quality of AI-generated images in models like Pony Diffusion. By providing a clear indication of image quality and enabling fine-grained control during the inference phase, these tags help in achieving more consistent and aesthetically pleasing outputs. As the development of AI models continues, refining these tagging systems and addressing their limitations will further improve the quality and versatility of AI-generated content.
If you like this article, please give it a thumbs up and share it. You can also try using my Pony Diffusion model for generation. Thank you.
〓〓〓〓〓〓〓〓〓〓〓〓〓〓〓〓〓〓 ★★★ FuturEvoLab ★★★ 〓〓〓〓〓〓〓〓〓〓〓〓〓〓〓〓〓〓
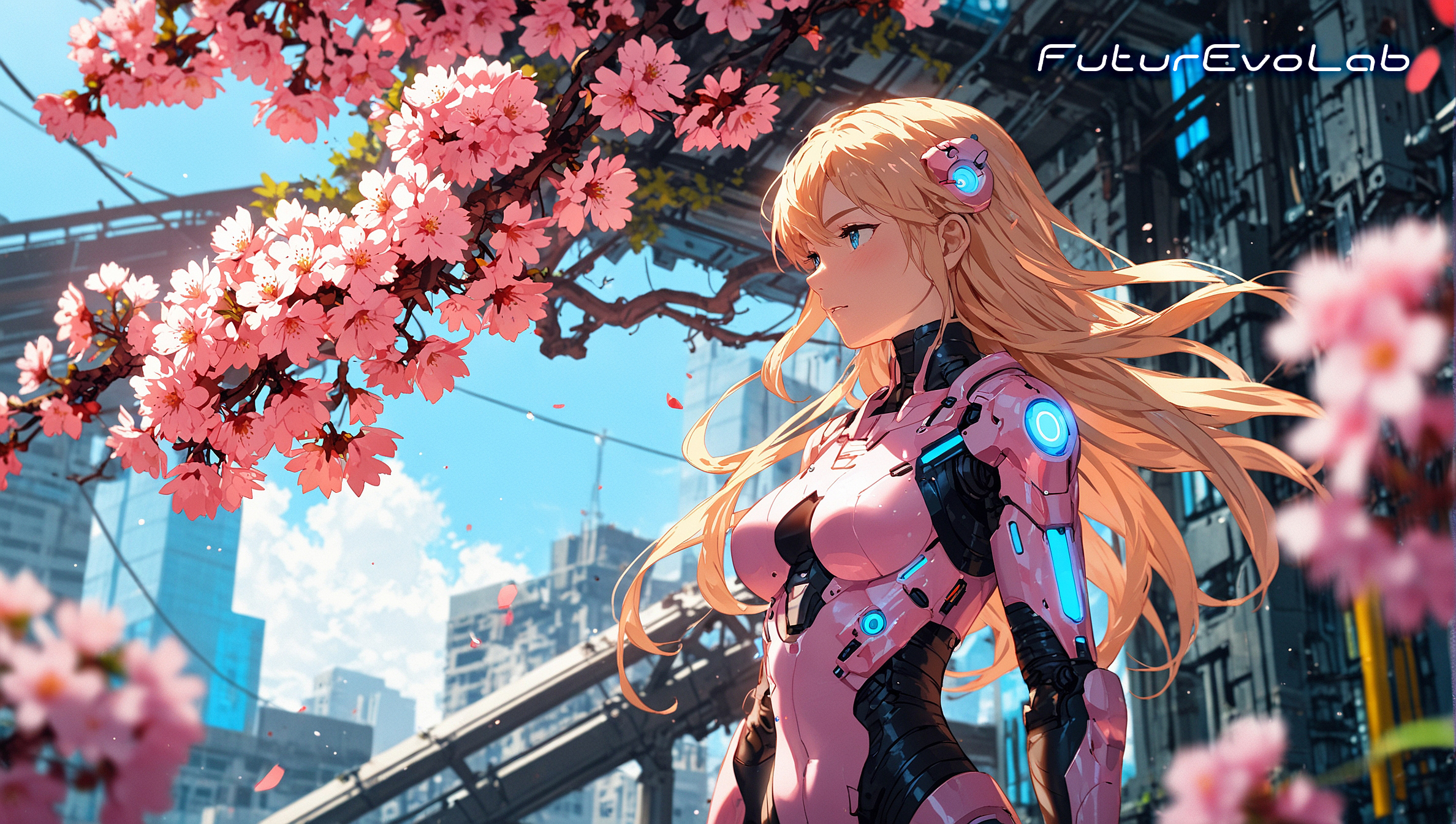
Welcome to FuturEvoLab! We greatly appreciate your continuous support. Our mission is to delve deep into the world of AI-generated content (AIGC), bringing you the latest innovations and techniques. Through this platform, we hope to learn and exchange ideas with you, pushing the boundaries of what's possible in AIGC. Thank you for your support, and we look forward to learning and collaborating with all of you.
In our exploration, we recommend several powerful models:
Pony XL (Realistic)
Pony XL (Anime)
SDXL 1.0 (Realistic)
SDXL 1.0 (Anime)
Stable Diffusion 1.5 (Realistic)
Stable Diffusion 1.5 (Anime)
By leveraging these models, creators can generate images that range from hyper-realistic to vividly imaginative, catering to various artistic and practical applications.
〓〓〓〓〓〓〓〓〓〓〓〓〓〓〓〓〓〓 ★★★ FuturEvoLab ★★★ 〓〓〓〓〓〓〓〓〓〓〓〓〓〓〓〓〓〓